Preventing and Accounting for Missing Clinical Trial Data for FDA Drug Approvals. Part 2: Analysis
by Eric Connor
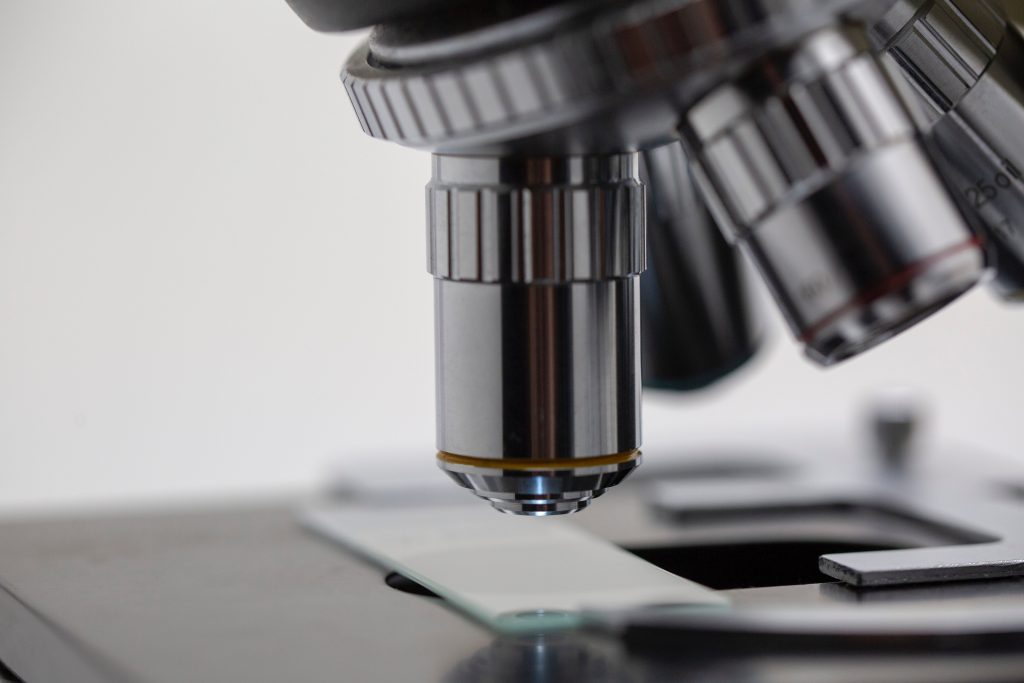
In Part 1 of this series, we discussed ways to prevent missing clinical trial data by incorporating key strategies in study design and patient follow-up. However, the reality of clinical trials is that having some missing data is inevitable, so we must also consider how to appropriately address the issue in the statistical analysis.
As the FDA becomes more stringent on how they expect sponsors to handle missing data in clinical trials, sponsors should carefully consider prespecified analyses that will support the robustness of the trial results. In order to understand the different approaches to account for missing data at the analysis stage, it’s important to understand types of missing data.
Types of Missing Data
We classify missing data in clinical trials as one of three types:
-
Missing Completely at Random (MCAR): missing data not related to the outcome or other variables in the dataset, e.g., site coordinator forgot to record key endpoint measurements on the case report form for a particular visit.
-
Missing at Random (MAR): missing data not related to the outcome, but other measured variables in the clinical study can account for what is missing, e.g., the patient missed a visit due to an extended vacation, but the patient remained on the study treatment.
-
Missing Not at Random (MNAR): missing data related to the outcome, e.g., a patient had side effects from the drug and was more likely not to take the study medication and miss study visits.
If all the missing data in the study were MCAR, we could ignore them completely in the analysis without risk of bias. In practice, however, the reason for most missing data in trials is not random, so below are some approaches to analyzing missing data that are MAR or MNAR.
Analyzing Missing Data
There is no one-size-fits-all approach for analyzing missing data in a trial. Each trial will require its own considerations. For illustration purposes, let’s use a simple example of a randomized, double-blind, placebo-controlled trial for a product to treat hypertension. Let’s assume that the primary endpoint in this trial is reduction in systolic blood pressure.
Simple imputation methods used extensively in the past, such as last observation carried forward (LOCF) and baseline observation carried forward (BOCF), are now actively discouraged by the FDA. Their rationale is twofold: these methods don’t properly account for uncertainty in the missing values, and their underlying assumptions are typically not justifiable. For example, a patient who was randomized to active treatment discontinued the drug due to an adverse event before the primary endpoint was assessed and then did not come back for any additional study visits. With LOCF, we would impute the patient’s primary endpoint value with a blood pressure measurement taken while still on active treatment. This is clearly inappropriate and a likely overestimate of the treatment effect because we wouldn’t expect the improvement in blood pressure to continue after the treatment has been discontinued. BOCF, on the other hand, can be overly conservative and lead to an underestimate of the treatment effect. For example, it would not be reasonable to impute “no improvement in systolic blood pressure” for a patient who missed their primary endpoint visit due to a family emergency but had been an excellent responder to treatment at all other study visits. In general, these simple imputations do not fully reflect the variability inherent in missing data and can lead to biased estimates of the treatment effect.
The most common methods for handling missing data assume that data are MAR. These include mixed models with repeated measures (MMRM), analysis, and multiple imputation (MI). MI is a common choice as a first-line method for missing data since other predictors in the dataset (e.g., gender imbalance in missing data rates, the reason for dropout) can be incorporated into the estimation of the treatment effect. These methods produce estimates that appropriately account for uncertainty and allow for valid inference if the assumptions of MAR are met. However, the MAR assumption cannot be tested.
The most common analytic method for data assumed to be MNAR is pattern-mixture models (PMM). These models assume that the response for patients who drop out and/or discontinue treatment is different than patients who continue the study. In our example of the hypertension trial, a PMM could assume that (1) when patients are still on treatment, missing data are similar to the observed on-treatment values, but (2) when patients drop out, they tend to have values less favorable than observed data (e.g., blood pressure values return to baseline). Sensitivity analyses like PMM ensure that the treatment effect is robust to the range of reasonable assumptions for missing values.
Overall, any analysis with missing data should be scientifically justifiable and, for the purposes of an FDA advisory committee, easily understood by a broad audience. Clinical experts and statisticians should work collaboratively to ensure that preplanned analyses for missing data are statistically sound and clinically reasonable.
All analyses for missing data rely on assumptions that cannot be tested or confirmed, so the primary effort should be toward preventing missing data in the first place. Incorporating practical elements into the study design, paired with a commitment to minimize missing data during the clinical study, will provide the most robust evidence for device and drug approval.